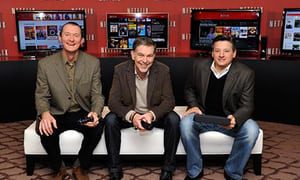
Does it ever feel like your computer has better taste than your friends?
Over the last couple of years, consumers have become accustomed to seeing the content habits of socially-connected peers shouted out from services like Spotify and The Guardian through channels like Facebook. Such recommendations are one flavour of the secret sauce of “discovery” that keeps users on board by continuously introducing them to new material. And these social referrals have already helped digital media services grow their audiences.
But, after a consumer backlash against Facebook’s noisy “frictionless sharing”, The Guardian ended the practise, while Spotify is now emphasising consumers’ new ability to derive their own recommendations from in-app intelligence and from the curators they choose to follow. Suddenly, socially-produced content recommendations seem a very blunt instrument to accomplish a very subtle goal, and this has left the floor open for more accurate, personalised suggestions to come from a different source – algorithmic engines.
“We create what we call a relevance map between content items like TV programmes in our database,” says RedBee Media chief technology officer Steve Plunkett, whose RedDiscover software engine is used by online video operators to recommend videos to viewers. “Each item is linked to other items like nodes in a network and those links are formed and weighted based on data that we collect or have access to.”
For a digital media service, linking these nodes in the right recommendation recipe can substantially grow consumer engagement. So important is the optimum engine that, in 2009, Netflix gave a $1 million competition prize to an outside group that successfully helped it improve its in-house CineMatch recommendations software by just 10%.
The effort has paid dividends. “Over 70 percent of what our members watch is based on our recommendations,” Netflix spokesperson Joris Evers says. “The more a member watches Netflix, the more likely they will stay a member longer.”
So what are the ingredients of a good recommendation?
“The more data, the better,” according to Rhett Ryder, CEO of Bath-based The Filter, which powers recommendations for BT Vision, NBC and others. “We ingest data about the digital content – ie. movies, TV, music, games, apps or books – as well as consumption data from millions of users to create a mathematical model that works out the strength of connection between every piece of content in the database. This connected model is based on millions of data points.”
While much of this may seem to borrow targeting tactics from online ad networks, which serve relevant ads by storing data about users’ browsing habits across the internet, content recommendations typically rely on breadcrumb trails left by users within an individual media service alone.
“Your viewing activity is the most important signal to us when it comes to generating recommendations,” according to Netflix’s Evers. The video service also produces recommendations based on viewers’ own ratings but: “We used ratings a lot more when we focused on DVDs in the US, since we had much less input from actual viewing activity for DVD.”
The ideal outcome introduces users, who may have already watched or read an on-site movie or story, to attractive next content from the same catalogue, keeping them plugged in for longer.
“Publishers’ prime responsibility is to increase the second click,” according to Stephanie Himoff, UK managing director of Outbrain, which helps online news outlets tantalise readers with suggestions of what to read next.
Outbrain has helped The Express website increase internal clicks by 12.8 percent, Himoff says: “An algorithm is based on other content that the reader has previously read, not just on the one article. This means that recommendations are highly personalised.”
If content services already hold all the necessary data that makes up a recommendation, why don’t they mix it together for themselves?
“In practice, these companies are content specialists, not data specialists,” says The Filter’s Ryder. “Netflix don’t use a third party because they have had a team and a series of algorithms entirely dedicated to recommendations for more than seven years. But most other content services have failed to make this investment.”
For anyone considering implementing a recommendation system, RedBee’s Plunkett advises: “Be very clear about what the recommendation is trying to achieve and always consider the user first.” The ideal scenario is to hold lots of consumption data, lots of context points and good-quality, consistent metadata, according to Ryder.
Ultimately, recommendation can be not just algorithmic but also social and produced by good old-fashioned human editors. So which methods drive most engagement?
The Filter’s Ryder says: “In one personalised app service, we measured the engagement and conversions for each of these three areas. The editorial section had the highest views, but the social and personalised sections had the highest conversion in terms of clicking through to purchase.” Purchase conversion was 18 percent from personalised recommendations against 12 percent from editorialised.
So is the writing on the wall for human channel editors, or for online journalists who have long manually added related stories to their pages? Outbrain’s Himoff says: “A journalist can’t know what every individual user is interested in.”
But RedBee’s Plunkett, whose RedDiscover engine is the result of acquiring TV Genius in 2011, adds: “An algorithm could be used by an editor to help find relevant articles that they can then choose from, or it could be used to link to other articles in different areas based on what reader actually did.
“Thankfully for our species, human and social curation are still very important. The optimal approach is, therefore, a combination of human expertise, knowledge and intuition combined with the massive number-crunching and pattern-matching capabilities of the machine.”