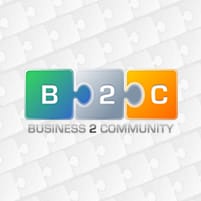
You can’t spend an hour at a tech event without encountering someone espousing the virtues of “Big Data.” Everyone wants to benefit from it – yet not everyone understands exactly what it is. Worse, the reality is that still fewer people are able to participate — yet.
For the uninitiated, Big Data is a quest to derive meaning from copious information by processing data, of whatever kind, in volumes that are so large or ways that are so intensive that it becomes difficult to process using traditional data processing applications.
One early application of this mysterious concept at work, which I used in a previous life as an investment analyst, is the software run by financial traders to crunch tens of thousands of news reports, market results and other unstructured content to rate the mathematical prospects of investment assets.
Now businesses of all kinds are being sold on the promise of turning data into gold. But, I’m sorry to report, we’re not there yet – and it may take years before the reality catches up with the hype. Here’s what to keep in mind:
1. Big data projects could be very expensive
We can all thank for putting powerful machines in the palms of our hands – but the alchemy required to mine gold from data is of a different order altogether. Big Data processing requires serious computer power – we’re talking clusters of computers.
Companies don’t own this hardware, they rent it – but renting processing time from vendors can start from $1,000 a day; Big Data projects from start to finish can start from around $1 million considering all the costs (data scientist, maintenance, etc).
This prices small- and medium-sized enterprises out of the game, and is a big cash drain on even larger firms.
2. Big data algorithms can’t solve all company’s problems
The actual statistical and computational models used in “big data” are more fitted to solve some kind of problems better than others. They are extremely good to find correlations between data sets, but they can’t understand if these correlations make sense.
For instance, when an algorithm encounters 5,000 references to “cat,” does it really know how to conceptualize a cat? When it processes the phrase “I saw her duck,” does it think the girl ducked – or that she showed off her feathered friend?
These are problems scientists are still working on – and it will be five to 10 years before businesses can derive the kinds of magical Big Data benefits they are being sold.
Solution? Here’s what we can achieve today
These two problems mean commonly-touted Big Data tasks – like mass translation, which requires extracting data from tens of thousand of text documents and performing structured sentiment analysis on customer comment forms for marketing personnel, are not nearly as practicable as businesses are led to believe.
That’s why I say to companies that want to embark on the Big Data bandwagon – start with something where big data could work best.
At Kuldat, for instance, we have decided to use big data to focus on tasks that are better performed by computers rather than humans , which gives our customers a real advantage when they run requests through out platform.
One example: big data platforms are a great tool for marketing and sales teams gain the competitive advantage by combing the Internet for valuable information they can collect, analyze, and connect to the bigger picture, such as new articles about target industries and the latest info about their competitors’ moves.
Keep in mind … this is only the beginning
Despite all the unsubstantiated noise out there about Big Data, we are at a very exciting time in its development . Big Data today is like computing in the 1950s , when machines were prohibitively expensive and deemed unnecessary for large-scale ownership.
Just like most people now own a computing device, all businesses will soon have access to their own big data platforms. But for now, we must set aside the overwhelming impossibilities of Big Data scale and simply get started today with what we CAN do before we can reap the greater benefits of tomorrow’s data revolution.